Project Overview
A biomedical researcher stares at their screen, frustrated. The tool they rely on to analyze research trends is clunky, difficult to navigate, and unable to handle the datasets they need. They waste time manually filtering information, struggling to find meaningful patterns.
As the lead UX/UI designer, I set out to solve these challenges, redesigning a data visualization tool for the National Institutes of Health (NIH) researchers, government agencies, and the public. The goal was to create an intuitive, scalable, and AI-powered solution that improves data discoverability, usability, and accessibility.
Key Outcomes:
72% faster data discoverability
30% reduction in onboarding time with an interactive walkthrough
Increased engagement through improved filtering and UI clarity
Enhanced accessibility compliance, ensuring equitable access
Before and after comparison
Understanding the Problem
The existing tool made it difficult for researchers and analysts to explore large datasets efficiently. Users faced several key challenges:
Inefficient Data Exploration: Users struggled with large datasets and lacked advanced filtering capabilities.
Lack of Intelligence: Data was grouped by extracted keywords rather than meaningful relationships.
Complex UI & Steep Learning Curve: The interface required excessive manual effort, frustrating users and slowing adoption.
Accessibility Barriers: No alternative views for visually impaired users.
Users needed
A tool that simplifies data exploration and surfaces relevant insights faster.
Smarter grouping and filtering to reduce manual work and encourage new data linkage discovery.
A UI that is intuitive and easy to learn, without extensive onboarding.
Inclusive accessibility features to support all users.
(Recommended Graphic: Persona card illustrating a primary user type, including their goals, frustrations, and needs.)
Existing data visualization tool
Drill-down progression
Research & Discovery
To design a solution that met real user needs, I led an in-depth research phase, combining user research, competitive analysis, heuristic evaluation.
Request for Information (RFI) Insights
To ensure the redesigned tool met real user needs, I drafted the initial RFI and worked with the team to refine it. The response was overwhelming—more than 6,850 participants shared their insights, providing a clear roadmap for improvement.
From the data, three top priorities emerged:
Ease of Navigation – Users wanted an intuitive interface to explore data efficiently.
Reliable Data – Trust in data accuracy and integrity was a top priority.
Analytical Support – The tool needed to help users derive insights, not just display raw data.
User Research
After identifying key prospective users garnered from the RFI, I conducted 13 user interviews with internal and external users, including researchers, analysts, and NIH stakeholders. The goal was to identify pain points, gather feature requirements, and uncover opportunities for improvement. Key findings included:
Difficult Data Exploration: Users struggled to refine and filter large datasets efficiently, making analysis cumbersome.
Lack of Intelligence: The tool grouped documents by extracted keywords rather than uncovering deeper relationships, limiting meaningful insights.
Usability Barriers: The interface was unintuitive, requiring steep learning curves and excessive manual effort.
Limited Output & Accessibility: A 5K document cap restricted data exploration, and there was no alternative format for visually impaired users.
Heuristic & Competitive Analysis
To align the tool with best practices, I conducted a heuristic evaluation and competitive analysis of industry-leading data visualization platforms, including:
Carrotsearch FoamTree- – Effective hierarchical clustering inspired better topic grouping.
D3.js.- Demonstrated the power of interactive, flexible filtering.
Findings from these analyses confirmed the need for improved discoverability, enhanced filtering, and better accessibility compliance.
(Recommended Graphic: Annotated screenshots of competitor tools, highlighting best practices that informed design decisions.)
Poor discoverability
Defining User Needs
Building on the research, I developed persona groups to represent key user segments and their needs. These personas helped guide design decisions by ensuring the visualization tool aligned with real user behaviors, challenges, and goals.
Ideation & Design
I collaborated with the team to explore and refine solutions through multiple design iterations. I began with hand-drawn sketches to quickly visualize ideas, then transitioned to low-fidelity wireframes to map out structure and functionality. As concepts evolved, I created mid-fidelity mockups, incorporating feedback to improve clarity, interactivity, and usability.
Wireframing and Prototyping
Design progression from sketch to prototype
Key Design Iterations Based on User Feedback:
Step-by-Step Walkthrough: Designed an interactive guide that auto-initiates for first-time users and can be relaunched anytime.
Step-by-step tool walkthrough
2. Expanded User Guide: Created detailed documentation to improve onboarding.
3. UI Clarity Enhancements: Adjusted labeling, hierarchy, and interaction cues for better discoverability.
4. Visual Aid for Complex Interactions: Collaborated with analysts to create a clear, intuitive representation of the tool’s logic.
Visual Aid
Users responded positively to these refinements, particularly the guided step-by-step walkthrough, with one noting:
“The interactive walkthrough made it so much easier to get started. I was able to understand the tool’s features right away, and I love that I can revisit it anytime for a refresher”
These improvements significantly reduced cognitive load, improved interpretability, and made the tool more accessible to new and existing users.
After implementing key design refinements, I conducted further user testing to validate improvements and ensure the visualization tool met user needs.
User Interviews & Testing
I conducted 8 additional user interviews to gather feedback on the revised interface, focusing on usability, comprehension, and efficiency.
To fine-tune UI decisions, I performed A/B testing with the internal team, comparing different design variations to determine which layouts, labeling strategies, and interaction patterns were most effective.
Validation & Refinement
Design iterations
Final Solution & Impact
Final Solution: AI-Powered Topic Explorer
After multiple iterations, research-driven refinements, and user-centered enhancements, the final visualization took shape.
Final visualization with improvements
The redesigned tool transforms how users explore and analyze data, offering tangible improvements:
Flexible Exploration: Users can browse an entire dataset without a predefined search, eliminating the need for exhaustive keyword lists. Alternatively, they can perform a search first and see results grouped into meaningful topic clusters.
Increased engagement: Users found navigation more intuitive.
Adaptive Display Levels: Most appropriate display level is automatically selected based on search results. Users can adjust the granularity by changing the "Display Level", choosing broader or more specific topic groupings.
ML-Driven Grouping: 72% faster data discovery through AI-powered topic groupings, which organize results by topic for quick interpretation.
Analyst-build topic map vs. automatically generated AI groupings
Interactive walkthrough: Contributing to a 30% reduction in onboarding time.
Data-Driven Visual Enhancements: Heat mapping (shading) reflects variations in a selected metric (e.g., percent early phase), allowing users to identify patterns at a glance.
Customizable Filters: Users can refine datasets dynamically to extract relevant insights.
Accessible Table View: To ensure inclusivity, I recommended and implemented an alternative tabular view of the Topic Explorer, allowing visually impaired users to access structured data beyond the graphical visualization.
Accessible table view
Impact & Key Challenges
Users praised the tool’s intelligence, flexibility, and clarity, with key feedback including:
“The AI-generated topic labels make it much easier to understand the dataset at a glance.”
“I appreciate being able to explore data without having to know the exact right keywords upfront.””
“The accessible table view makes this tool much more usable for a broader audience.”
Key Challenges
Takeaway
This project reinforced the power of user-centered design, iterative testing, and accessibility-driven innovation in data visualization. By focusing on efficiency, usability, and inclusivity, I transformed a frustrating tool into an intuitive, insight-driven experience for researchers and analysts.
Final visualization
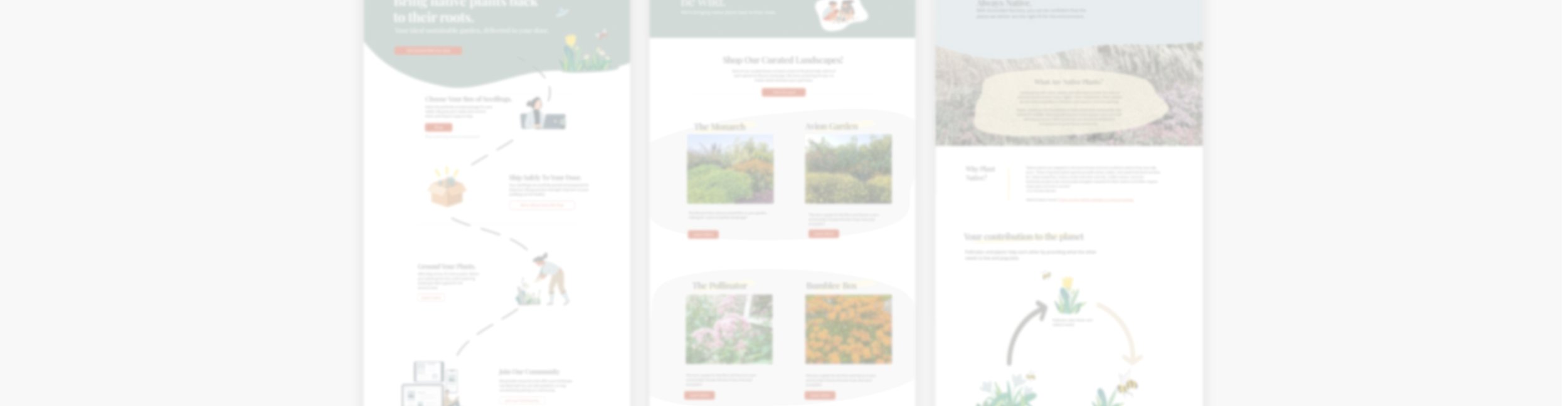
IssueVoter
UX Designer, UI Designer